81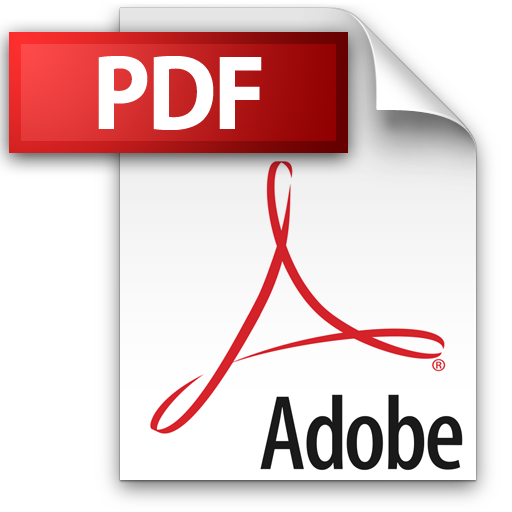 | Add to Reading ListSource URL: trec.nist.govLanguage: English |
---|
82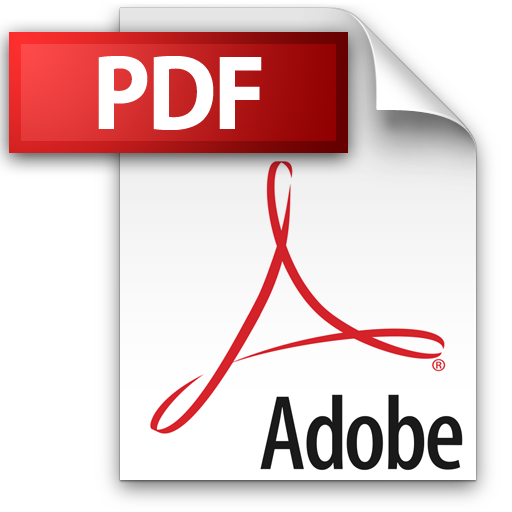 | Add to Reading ListSource URL: research.microsoft.comLanguage: English - Date: 2014-04-14 03:27:42
|
---|
83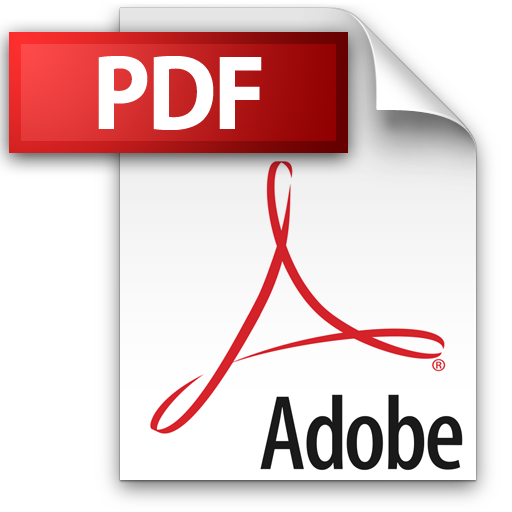 | Add to Reading ListSource URL: trec.nist.govLanguage: English - Date: 2012-02-03 09:41:35
|
---|
84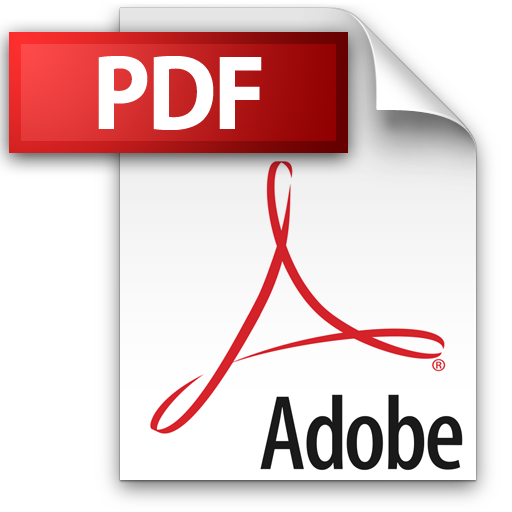 | Add to Reading ListSource URL: trec.nist.govLanguage: English - Date: 2012-02-03 09:41:33
|
---|
85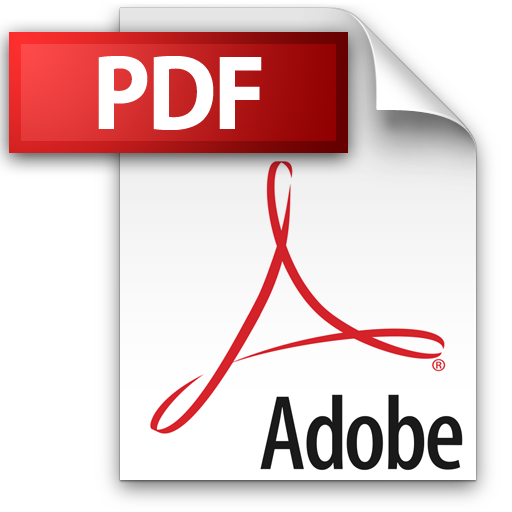 | Add to Reading ListSource URL: www.dcs.gla.ac.ukLanguage: English - Date: 2012-08-21 14:57:59
|
---|
86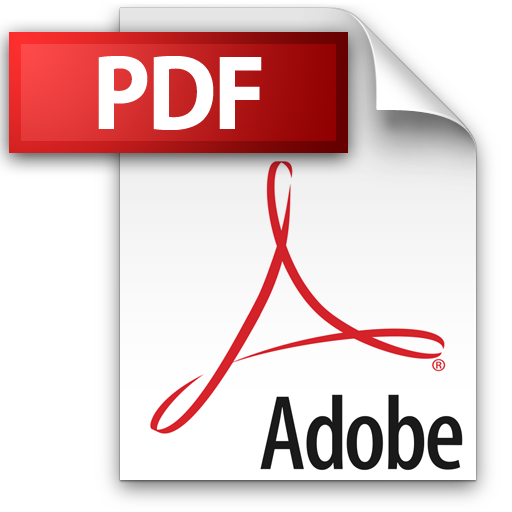 | Add to Reading ListSource URL: trec.nist.govLanguage: English - Date: 2012-02-03 09:41:30
|
---|
87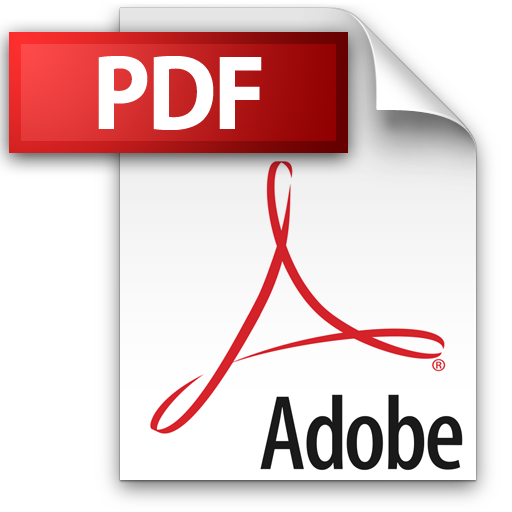 | Add to Reading ListSource URL: www.wsdm2009.orgLanguage: English - Date: 2009-04-14 09:42:11
|
---|
88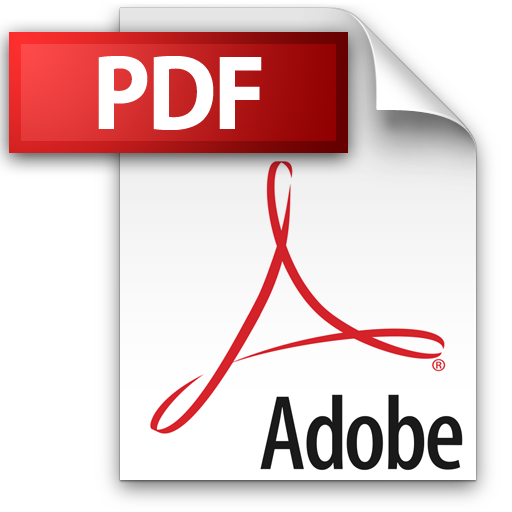 | Add to Reading ListSource URL: www.wsdm2009.orgLanguage: English - Date: 2009-04-14 09:41:44
|
---|
89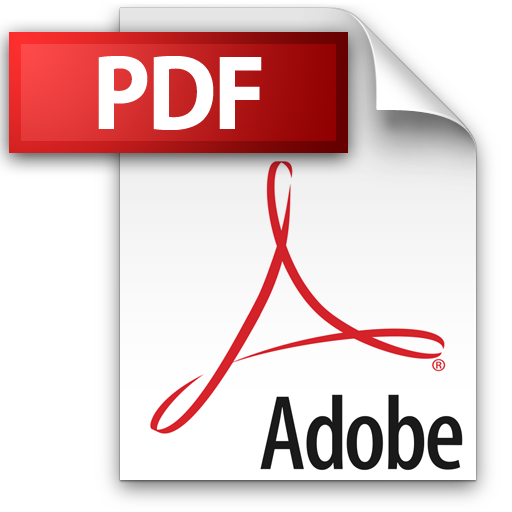 | Add to Reading ListSource URL: www.thuir.cnLanguage: English - Date: 2009-06-27 05:08:51
|
---|